Introduction
It is said that to explain is to explain away. This maxim is nowhere so well fulfilled as in the area of computer programming, especially in what is called heuristic programming and artificial intelligence. For in those realms, machines are made to behave in wondrous ways, often sufficient to dazzle even the most experienced observer. But once a particular program is unmasked, once its inner workings are explained in language sufficiently plain to induce understanding, its magic crumbles away… (Weizenbaum, 1966, p. 36)
Much like Joseph Weizenbaum’s attempt to break the magic of artificial intelligence (AI), as illustrated in this quote from his 1966 paper, this article addresses the current magical allure of AI in K-12 education in an attempt to unmask specific aspects related to the promises of the automation and augmentation of teachers. Rather than exposing the inner workings of AI technology, we focus on the teachers’ work that takes place behind the scenes as AI is being introduced into K-12 classrooms.
AI in education is already an integral component of the global education industry (Komljenovic & Lee Robertson, 2017) and is trending in the discourse of contemporary education (Tuomi et al., 2023). However, it has a long history, with research on AI in education dating back almost fifty years (du Boulay, 2022; Holmes et al., 2020; Zawacki-Richter et al., 2019). Contemporary AI in education is based on a data-driven approach, often incorporating machine-learning techniques for algorithmic knowledge extraction and computational decision-making (Holmes & Tuomi, 2022), and overlapping with the field of learning analytics (LA) (Buckingham Shum & Luckin, 2019; Siemens, 2013). The research on AI in education and LA has developed along two complementary strands which can be broadly associated with the concepts of automation and augmentation (Molenaar, 2022; Sperling et al., 2023).
Automation refers most commonly to the development of intelligent tutoring systems, virtual assistants and automated marking designed for classroom use (Holmes & Tuomi, 2022), but also to facial recognition systems and school monitoring (Selwyn, 2022a). While the automation of teaching is a continuous topic of debate (du Boulay, 2019; Selwyn, 2019a), here, different AI technologies are intended to handle tasks that would typically require teacher involvement, with the goal of automating or completing these tasks independently. Thus, this approach can also be understood as a replacing perspective (Cukurova et al., 2019), implicitly suggesting that not only teaching but also teachers’ educational judgement can be optimised by replacing teachers with AI (Selwyn et al., 2023). Not only does the automation of teaching as introduced through applications, software and hardware reshape pedagogical practices (Popenici & Kerr, 2017), it also renders the pedagogical work more machine-like (Perrotta et al., 2021).
The second strand of research––the augmentation of teachers––is all about enhancing teachers’ comprehension and bolstering their capabilities as educators through the use of LA systems, which are increasingly intersecting with AI and are aimed at measuring, predicting and understanding students’ learning processes (Siemens, 2013). What are termed ‘learning analytics dashboards’ (LADs) are perhaps the most common manifestation of this trend, and these can be found in, for example, learning management systems (LMS) and other educational technologies (Matcha et al., 2020). Teacher-oriented LADs are commonly described as interfaces with aggregated data about students and their progression visualised in ways in which relevant information can be perceived at a glance (Schwendimann et al., 2017). Their purpose is to assist or ‘nudge’ (Park & Jo, 2019) teachers in gaining a comprehensive understanding of their courses or specific tasks, evaluating their teaching methods and identifying students at risk and in need of individual attention (Molenaar & Knoop-van Campen, 2019; Verbert et al., 2014). Such approaches mark a change from the automation-replacement viewpoint to what can be referred to as an augmentation-reinforcement perspective (Cukurova et al., 2019; Molenaar, 2022). Here, an essential aspect is gaining insight into the functions of hybrid teacher-AI usage scenarios and envisioning complementary roles for both students and teachers alongside AI systems. The latter is also referred to as ‘hybrid intelligence’ (Akata et al., 2020) and speaks to the synergistic potential of human intelligence and AI (Dellermann et al., 2019).
Despite the increased, albeit uneven, adoption of AI and LA in K-12 education (Ferguson & Clow, 2017), the evidence base for their positive effects on education remains shrouded in ambiguity (Holmes & Tuomi, 2022; Viberg et al., 2018). While there exists increasingly comprehensive and critical analyses of the power structures, ethical dimensions and educational implications of AI and LA technologies in education (e.g., Knox et al., 2020; Lupton & Williamson, 2017; Perrotta & Selwyn, 2020; Perrotta & Williamson, 2018; Selwyn, 2019b), we still know very little about the practical implementation of AI technologies in K-12 classrooms, particularly regarding how they influence teachers’ practices (Williamson & Eynon, 2020). This paper addresses this research gap, drawing on two ethnographic studies conducted in Swedish K-12 classrooms. By exploring the enactments of teachers and two different AI technologies: (1) a machine-learning-based teaching aid, and (2) a LA-integrated LMS, the aim is to demonstrate how automation and augmentation might emerge in situated practices. We address this aim through the following research questions:
1. How does the automation of teaching unfold in teachers’ practices?
2. How is augmentation of teaching played out in teachers’ practices?
This examination of automation and augmentation in classrooms may contribute to a nuanced understanding of the multifaceted dynamics surrounding the introduction of AI in Nordic educational settings and beyond. In the remainder of the paper, we outline the theoretical and methodological approaches employed before presenting four distinct actions displaying how automation and augmentation unfold in teachers’ work. We conclude by discussing these findings in the light of teachers’ hidden work and potential dilemmas emerging from the growing presence of AI and LA in classrooms.
A relational epistemological lens on automation and augmentation
To elucidate enactments within the context of AI and LA in classrooms, the paper adopts a sociomaterial perspective, a theoretical framework rooted in relational epistemology (Bearman & Ajjawi, 2023; Dépelteau, 2013; Latour, 2007). Our conceptualisation of such enactments posits them as complex socio-technological phenomena characterised by dynamic interactions between human and non-human actors in the messy, multifaceted and energetic practices of classrooms (Fenwick & Landri, 2012). The interactions, recognised as relational effects, shape actors’ actions within an educational ecology (a classroom). They influence and lead to mutual changes (Fenwick & Edwards, 2010). This theoretical approach underscores that AI in education—rather than being defined according to its technical configurations—should be understood according to how it effects and mutually shapes actions together with other actors in the ever-evolving nature of teaching/technology/educational practices (Law, 2004).
So, rather than merely defining AI in education, our research shifts its focus towards understanding the intricate process of how AI in education, in the context of automation and augmentation, is embodied in the teachers’ practices. This implies a study of enactments in the classroom, a process which is made up of many individual actors, causally connected, producing and interacting in tandem; a relationality that is treated as effects (Law, 2004). From this standpoint, nothing is truly self-organising or autopoietic (from Greek autós [αὐτός], self, and poíēsis [παραγωγή], to make or produce) in a classroom. Rather, everything is sympoietic (from Greek sún [σύν], with or together, and poíēsis [παραγωγή], to make or produce), which refers to a collective creation or organisation (Petersmann, 2021). Hence, something that may look like automation and/or augmentation due to the introduction of new technology might, instead of the ‘auto’ and ‘augment,’ be more of a ‘sym,’ a ‘with,’ in which students, teachers and machines co-produce actions (Wagener-Böck et al., 2023, p. 137). For this reason, inspired by Wagener-Böck et al. (2023), we elaborate further on their ideas and suggest that both automation and augmentation in educational practice should be understood as a collaborative endeavour, a ‘symmation.’ In the analytical work in this paper we therefore adopt the term symmation when studying socio-material/technical enactments in classrooms. This concept helps us to make sense of how enactments in classrooms contingently transform and leave space for collective modes of being, thinking and acting.
An ethnographic methodological approach
Methodologically, the theoretical framework rooted in relational epistemology advocates for an ethnographic approach, which means following actors with a focus on particular enactments: the mutual constitution of entangled socio-technical arrangements where bodies, voices, desks, algorithms, websites, sighs, chairs, apps, playfulness, weariness, languages, gazes, and much more produce and change everyone’s world (Fenwick & Edwards, 2010; Wagener-Böck et al., 2023). A relational understanding involves different forms of researcher subjectivity, the incorporation of diverse methods for data generation and an analytical approach that permits the identification of patterns and configurations, allowing for unforeseen readings of and engagements with the data (cf. St. Pierre & Jacksson, 2014). As outlined in the following sections, this paper is based on two distinct ethnographic studies carried out in Swedish K-12 classrooms (cf. Sperling et al., 2022, 2023), using various methods and an analytically sensitive, transparent engagement with the data (Jackson & Mazzei, 2013).
Study I: A machine-learning-based teaching aid
A nationally co-funded innovation and research project about AI in mathematics teaching (2020–2021) in Sweden, involving 22 teachers and over 250 primary and lower secondary school pupils, constituted the context of Study I. The project’s focus was to explore the potential of a machine-learning-based teaching aid in mathematics known as the AI Engine and determine whether it could automate specific aspects of arithmetic instruction. The AI Engine’s learning content is comprised of different training modules covering arithmetic exercises (addition and subtraction) in the number intervals of 1–10, 1–20 and 1–100, as well as multiplication table exercises. The modules were tried out in 22 classrooms, encompassing pupils aged 8–9, 11–12 and 14–15. This implementation took place over the course of two six-week interventions, during which the pupils exercised with the AI Engine for ten minutes, three times a week. Fieldwork was centred around the interactions between the project team (representatives from a local school authority, a teaching-aid company and education researchers), the teachers and the pupils in the 22 classrooms and the AI Engine. During fieldwork, the second author was an employee of the local authority and already involved in the project, which was in the process of applying for funds, and was a member of the project team. Assuming the ‘insider’ role as a researcher is likely to have provided access to a more in-depth understanding of the project’s development while, at the same time, this role might have led to certain things being taken for granted and other things going unnoticed (cf. Sperling, et al. 2022).
Data production
Data were produced with the aim of exploring enactments of AI technologies and teachers. Due to the COVID-19 pandemic, which hindered much of the planned fieldwork, different methods were employed. The produced data included field notes from four classroom observations in Years 2 and 5 that took place during the second and third week of the first of the two interventions. Seven online, semi-structured interviews with three experienced lower secondary school teachers and four representatives from the project team (a project manager, a development teacher at the local authority, a teaching-aid author and an education researcher) were carried out between May and June 2021. The interviews, ranging from 45 to 90 minutes, were each recorded and then transcribed. These interviews were primarily seen as a way to capture accounts of enactments that were not directly observable (Mazzei, 2013).
Study II: An AI and LA-integrated LMS
The fieldwork of the second study spanned over two years (December 2020–December 2022), focusing on the development and co-design of an AI and LA-integrated LMS targeting Swedish K-12 students. This LMS was designed for lesson planning, management and the provision of analytics related to student attendance, performance, motivation, enjoyment, and well-being. The selection of the LMS was based on an extensive review of educational technologies featuring AI that had been implemented in Swedish K-12 schools and beyond. During the period of the study’s fieldwork, the LMS was co-designed with teachers in two K-12 schools: one was a combined primary and lower secondary school with over 1,200 pupils aged 7–15, while the other was an upper secondary school specialising in technology, with approximately 450 students aged 16–19 (cf. Sperling, 2023).
Data production
In this study, data were produced to explore enactments of AI technologies and teachers. The materials derive from a range of different methods for data production in order to broaden the analysis: observations in the different spaces of these schools, formal and informal interviews with teachers, blog posts, webpages, YouTube tutorials and social media. Approximately 80 hours of classroom observations, observations during three co-design workshops and observations at a trade fair were documented using fieldnotes and anonymised photos. Thirteen in-depth interviews were held with seven primary, lower and upper secondary teachers from the participating schools, one principal and one representative from the local school authority, and three representatives from the EdTech company (a UX designer, a product developer and the company founder responsible for the LMS). The interviews ranged from 50 to 90 minutes and aimed to provide a comprehensive understanding of the co-design process and the implementation of the LMS from multiple perspectives.
Analytical procedures
The analytical procedures with the data from Studies I and II follow an abductive approach involving a systematic analytical procedure characterised by iterative movements between the empirical data and theoretical constructs, aligning with the overarching research aim (Lather & St. Pierre, 2013). To illustrate, multiple analytical sessions were conducted during this methodological process. This means that one of the researchers, the second author, meticulously examined the entirety of the collected data, including perusing fieldnotes and reviewing the transcribed interviews and photos, thereby ensuring a holistic and nuanced understanding of the entire dataset. By choosing not to divide the data between the researchers, a comprehensive oversight was achieved. Both researchers simultaneously engaged with relevant theoretical literature, fostering an openness to novel associations and first interpretations (Jackson & Mazzei, 2013). This collaborative approach not only broadened the scope of insights derived from the literature but also facilitated dynamic discussions, enabling an exploration of potential connections between theoretical concepts and the data. Then, through an iterative dialogue between produced data and theory, the identification of significant elements related to the research questions was facilitated. This involved discerning particular words, phrases and fragments of digital interfaces present in the classrooms (Stafford, 2001). In essence, certain situations depicted in fieldnotes, photos, interview transcripts or other data samples assumed heightened significance, warranting further investigation (MacLure, 2013). These situations were everyday occurrences and represented situation-specific manifestations that encompassed a spectrum of enactments in the classrooms. They involved not only teachers, students, and specific AI technologies but also encompassed computers, desks, bodies, textbooks, curricula, and classroom spaces, among other factors. The significant elements that drew our attention became focal points for our subsequent analyses. That means the selected situations were crafted into cohesive events based on fieldnotes, photos, and interview transcripts. The data encapsulated within the events was translated into English. Finally, the analysis of these events was conducted by employing symmation as an analytical concept (see also Wagener-Böck et al., 2023). Symmation was instrumental in examining the ‘automation of teaching’ and/or the ‘augmentation of teachers,’ as articulated within the events and supportive in distinguishing various facets of teachers’ practices.
Four enactments of symmation
In this section, we present four events1 based on the fieldnotes, photos, and interview transcripts mentioned earlier. Each event highlights symmation: how in fact teachers’ (hidden) work is needed for automation and augmentation to emerge. We illustrate how this work is characterised by teachers’ adaptations, experimentations, compensations, and confirmations.
Automation unfolding in teachers’ practices
The first set of events was crafted from data produced in Study I, in which the machine-learning-based teaching aid in mathematics, the AI Engine, was introduced in primary and lower secondary school classrooms. The actions in these events were characterised by teachers’ adaptations and experimentation.
Event 1: Adaptations
The maths lesson for a group of 20 Year 2 pupils is drawing to a close, marking the onset of a 10-minute individual exercise with the AI Engine. The pupils sit in front of their computer screens and enter numbers in the free-pacing training module. The AI Engine displays the exercise 64–56 on several computer screens. The pupils type in their answers, upon which similar number combinations follow. Some pupils exclaim, ‘hey, this is too difficult,’ yet the classroom atmosphere is one of intense concentration and perseverance. The AI Engine keeps on delivering new exercises. The pupils assist one another and raise their hands to seek help from their teacher Elsa. She is in constant movement, circulating around the classroom and addressing each pupil who raises their hand. She keeps on instructing pupils that get stuck and makes sure that everyone has understood the strategies presented during the lesson and that are necessary to progress.
This event illustrates how pupils aged 8–9, along with their teacher Elsa and the AI Engine, co-produce automation. While the AI Engine is designed for individualised use and the pupils earnestly strive to solve the delivered exercises correctly, some pupils complain, seeking assistance from their peers and the attentive teacher. For automation to emerge, significant adaptation is required from Elsa, as manifested through the instructions specifically addressing the level of difficulty delivered by the AI Engine, as well as active monitoring and scaffolding during the 10-minute exercise. Rather than the AI Engine automating the work of Elsa by adapting the exercises to every individual pupil, Elsa must adapt her actions to the algorithmic decision-making for the exercise session to succeed. Thus, the co-production—symmation—where the teachers and pupils in collaboration with the AI Engine create the misleading illusion of automation. In the next event we highlight a slightly different symmation process where teachers need to experiment when faced with situations where the AI Engine does not deliver exercises as anticipated.
Event 2: Experimentation
Henry: This engine is great! It just keeps going. The exercises don’t really end. The issue, however, was that they couldn’t type the answer. And then there was this… what was the module that came after the ‘Number Chase’ called?
Interviewer: ‘Exercise more’? The one with a lot of exercises?
Henry: Yes, we worked with it. […] It was a bit too difficult for quite a few, but really great for those who are more proficient, as they were challenged. However, I told some pupils, ‘This looks a bit tough; you should go back to the “Number Chase” module.’ So, we experimented a bit.
From this event, which illustrates a conversation between Henry, a primary school teacher, and the researcher, we understand that Henry had to direct his pupils back and forth between different modules to help them accomplish exercises with the AI Engine. When some of the modules were not working as expected, he tried out new ones created as a response by the teaching-aid author to compensate for emerging errors. Henry recounted that these modules suited some pupils well but other pupils that struggled needed to be directed back to the old modules. This constant monitoring of which pupil was getting which exercise was demanded of the teacher to create the impression of automation. While Henry holds the belief that the AI Engine ‘just keeps going’ and can adapt to the pupils’ abilities, he gave an account of doing an extensive amount of work related to monitoring and personalising during the exercise sessions. Rather than just adapting, Henry needed to try out new modules and direct the pupils in a way that he described as experimental. Thus, his experimentation with various available modules, the pupils who complied with trying out different modules and the AI Engine performing ‘great’ in some cases and not at all in others, co-produced something that looked like automation, at least for some of the more proficient pupils. In reality, symmation necessitates considerable extra work, where the teacher, while trusting the AI Engine to work, needs to be attentive to the breakdown of the system and find different strategies for the pupils to train with the AI Engine. This work, as well as the pupils’ compliance with Henry’s experimentation, was entangled with the machine-learning algorithms of the AI Engine, in what may have looked like automation. A new module, ‘Exercise More,’ was created by the teaching-aid author to mitigate some of the encountered problems. While this module included more exercises, it proved effective primarily for the most proficient pupils. Other less-proficient pupils required redirection to the previous modules by the teacher.
In contrast to the narrative of AI in education as a time-saving measure, the first two events manifested how teachers’ adaptations and experimentation not only served as compensatory measures but also demanded significant—yet hidden—labour and effort. The enacted automation emerged as a messy and entangled symmation process, involving teachers, pupils, the technology and the entire learning environment.
Augmentation played out in teachers’ practices
The next two events pertain to Study II, in which the AI and LA-integrated LMS was collaboratively developed and tested by primary and secondary school teachers. The events illustrate how augmentation of teachers’ practices was enacted in classrooms as an effect of symmation. The enactments stemmed from the work of teachers and pupils in close conjunction with the integrated technology, in this case the LAD (Figures 1 and 2) and daily questionnaires assessing the pupils’ well-being, motivation, lesson satisfaction, self-reported learning and peace of mind. The outcomes of these questionnaires were showcased through vibrant visual representations and could be explored by the teacher at various levels of detail.
Event 3: Compensations
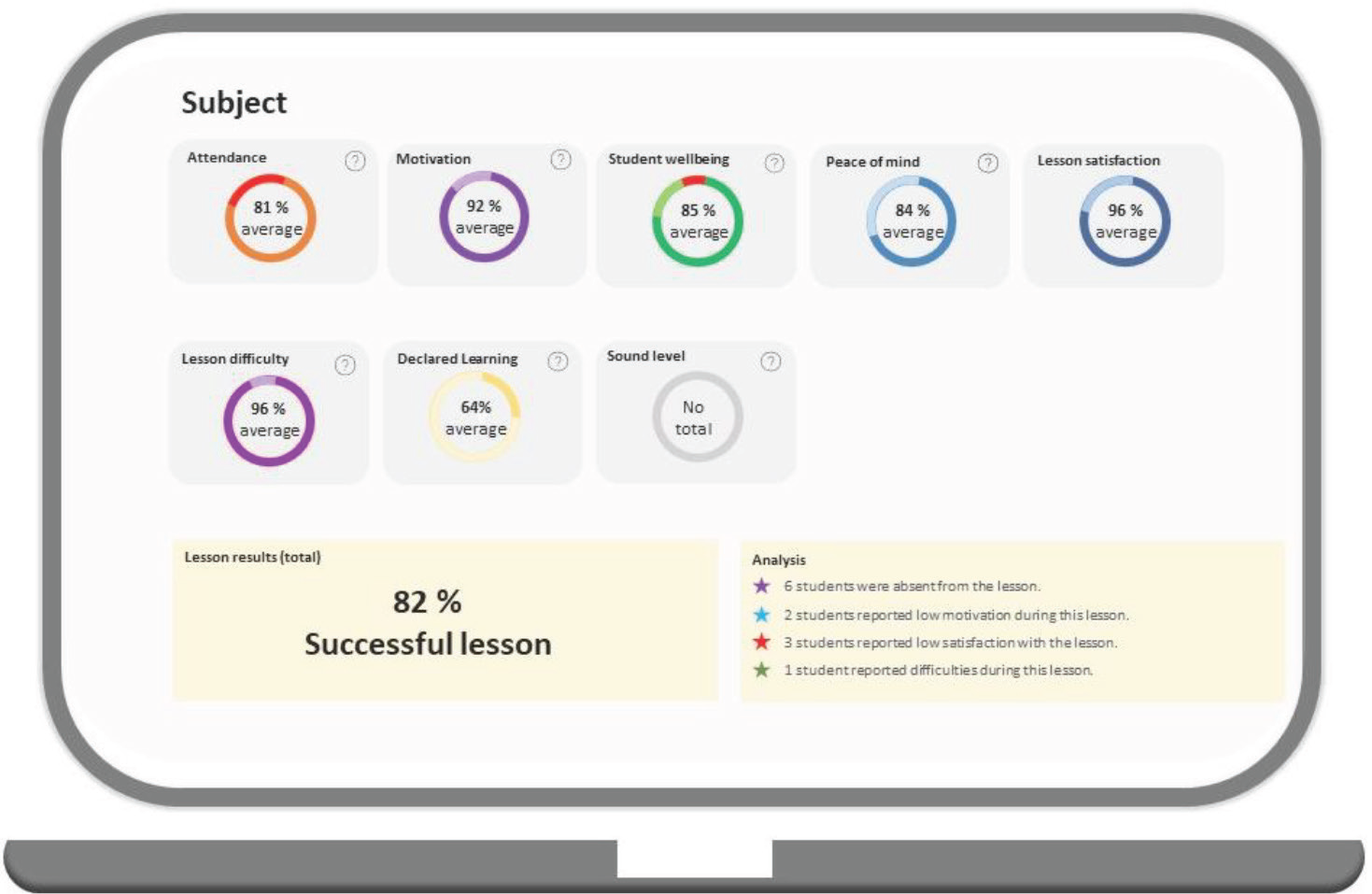
Alfred: So, here you can also see the analytics [referring to the LAD], and I find it really interesting. First, I notice that they [the pupils] have a very high motivation during the lessons, their attendance is extremely high and there’s a great level of focus. The lesson satisfaction level is 96%, but they [the pupils] still think that the degree of lesson difficulty shouldn’t be like, ‘it was very easy, and we just watched a movie’ because then you can achieve very high results. Rather, it’s about, and I talk a lot with them [the pupils], that they have to work hard and in a varied way, and that the right level is individual, not too easy but not too difficult either. […] So, for me, it’s a confirmation that the time I spend on analysis and conversations with the pupils, etc., also yields results.
Interviewer: But when you see this analysis [points to the stars in the corner with alerts on low score], what do you do?
Alfred: I usually check who it is, and there’s usually an explanation […] With low lesson satisfaction it can be like, ‘well, I already knew this, so I thought I hadn’t learned much’ […] So, they don’t always understand exactly what they’re filling in either, you know.
15 minutes later
Interviewer: Is there anything else you’d like to share, anything that comes to mind about the LMS, any concerns perhaps?
Alfred: Well, the concern is that we might rely too much on it [learning analytics] […] Numbers are one thing, but aspects like, ‘I have seen a pupil because I’ve seen their data,’ it’s not really the same. So, that’s a concern, that you must not forget that just because I’m sitting here at my place and the pupils are inside the system, then we are connected. But we are not, you know, the most important part is outside all of this, and one must never forget that.
In this conversation with Alfred, a primary school teacher, symmation—as opposed to mere augmentation—came into existence through the assembly of diverse visual elements, including the LAD, computers, the pupils’ compliance, (mis)interpretations of the questions and the teacher’s compensatory actions. At first, Alfred explained what he could tell from a specific view of the LAD that referred to a lesson a few months ago. He seemed satisfied with the figures displayed in the colourful pie charts, attributing the high average score for lesson satisfaction (96%) to his systematic use of the LMS and the work invested in interpreting the various visual elements of the dashboard (numbers, headings, pie charts, stars), both during and after class. Alfred’s work also entailed making sure that the answers his pupils, aged 10–11, provided through the automatically generated lesson questionnaires corresponded to his concept of effective teaching and learning. This demonstrates not only that Alfred needed to become involved in analysing the data but also that he had to prepare detailed instructions and oversee responses to ensure that the pupils answered in ways he deemed adequate. Thus, Alfred compensated by carrying out substantial work, such as teaching the pupils how to interpret (and respond to) the questions, to enable the augmentation to emerge. The co-production of augmentation—symmation—emerged from Alfred’s compensatory work together with the pupils’ compliance with the data collection and the data visualisations of the LAD. Symmation was also reflected in Alfred’s compensatory work as a response to the stars positioned in the lower right-hand corner of the LAD’s interface (Figure 1). The stars signify low scores that the teacher should ‘take action on,’ prompting Alfred to make sure that these numbers were not indicators of pupils falling behind or feeling unwell. However, drawing on his past experience, Alfred asserted that the stars were not necessarily indicative of a genuine problem but were, in most cases, indicative of the pupils misunderstanding the questions. His statement, ‘So they don’t always understand exactly what they’re filling in either you know,’ together with his compensatory work of producing the LA data contradicts the narrative suggesting that the gathered, analysed and visualised data helps teachers to act less on intuition and more on evidence. As illustrated in this event, Alfred needed to compensate for the limitations of the LAD by continuously instructing and monitoring the pupils, which also included disregarding responses resulting from pupils misinterpreting the questions. At the same time, the data analytics practice could impose different ethical dilemmas. For example, Alfred might have overlooked the possibility that the pupils, when questioned about their negative responses, might have hesitated to take responsibility for their answers. Thus, when contradicting the teacher’s knowledge about his pupils, the LAD still instilled doubt as to whether the pupils were truly being honest or not. The event, therefore, also highlights how the practice required Alfred’s reflexive understanding of the risks involved in translating pupils into data without having a close relationship with and prior knowledge of the pupils. This understanding underscores again how symmation, and not augmentation, is at play. Here, the teacher’s relational knowledge about his pupils’ well-being and progress compensated for the limited insights that the LAD provided. In a similar vein, the next and final event shows how symmation depended on the teacher Kim confirming the LAD with already existing knowledge.
Event 4: Confirmations
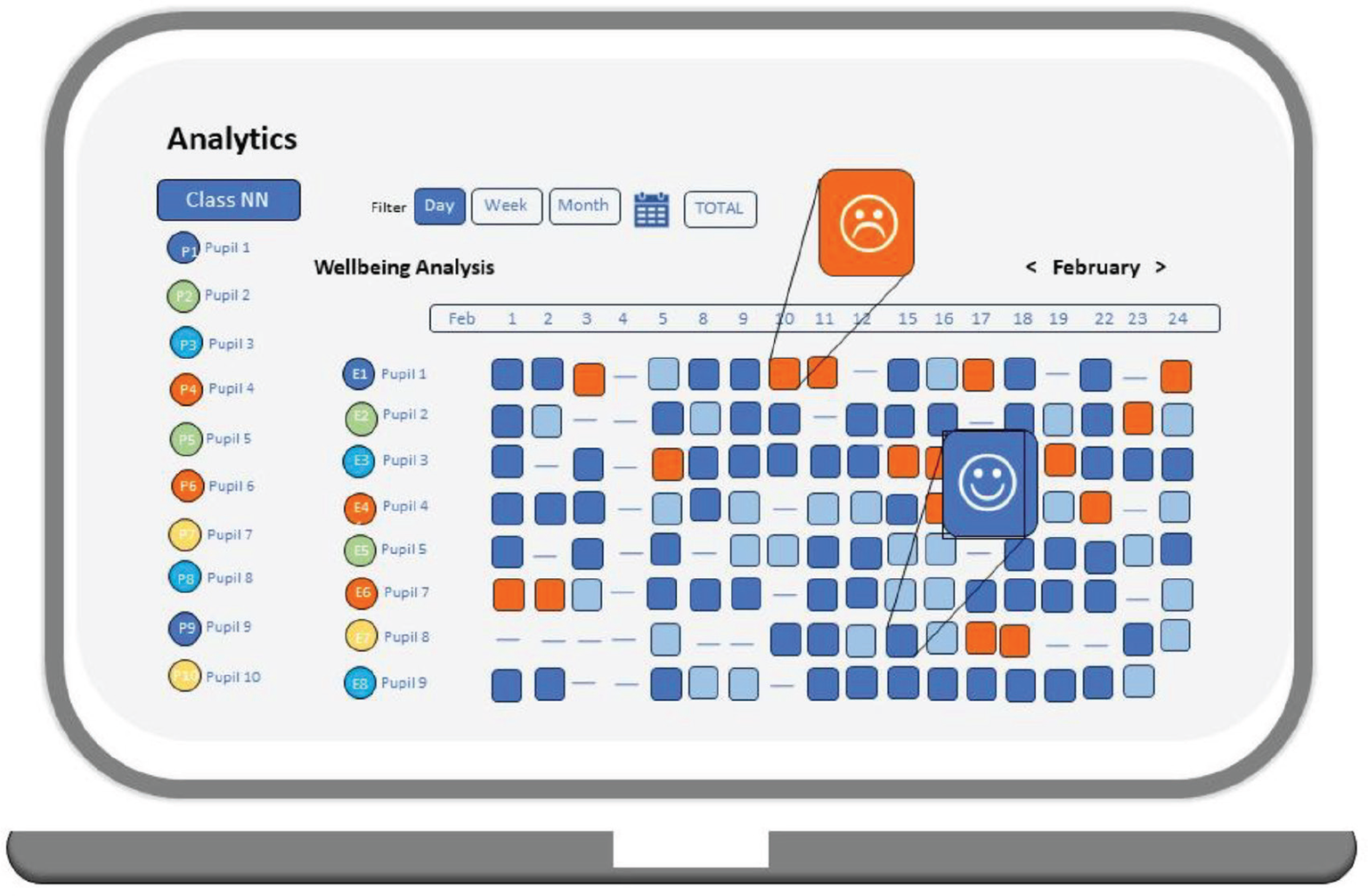
Kim: Because if you look at this list on reds…it’s not…the ones that have a lot of red, I’m not surprised that I see a lot of red there. Because I know that as I see them in the classroom too. But what I see in the classroom is also part of a gut feeling. Here, it is [points to the screen] visible. Here they have also put their… [does not finish the sentence].
Interviewer: Mmm. But you also said [earlier in the conversation] that some of them just check the boxes?
Kim: Well, some just check them, yes. But I don’t think everyone, I think it’s only a small group that checks, at least that is what I hope in the groups that we looked at. In those groups, only some of them check the boxes, mmm.
Interviewer: Right, and you know that because…?
Kim: Because I know who they are. Because I have seen them in the classroom, every week for almost a year […] I spend a lot of my class time walking around and talking to my students and seeing how they’re doing when they’re working. Mmm.
Interviewer: So you don’t really need this?
Kim: But it’s a good confirmation, to know that it’s not my gut feeling.
The red squares with emojis (Figure 2) seemed to play a key role in this conversation where Kim, an upper secondary teacher, rather than dismissing them confirmed their relevance. However, something that at first may have looked like augmentation, here represented by the LAD’s visual representation of the students’ responses, instead turned out to be more of a ‘sym,’ a ‘with.’ Here Kim needed to support the red emojis of the LAD, which are intended to be indicators of students not doing well. However, Kim appeared neither surprised nor troubled by their appearance, which may stem from the teacher’s observations in the classroom that some students, aged 16–17, merely checked the boxes. This suggests that the teacher compensated for the different source of errors impacting on the data by constantly paying attention to what was happening in the classroom. Without this work, so significant for the teaching profession, Kim would have struggled to comprehend the importance of visually representing the students’ well-being. Thus, in this example, symmation means that the teacher’s knowledge about the students’ well-being, cultivated through time spent in the classroom walking around and talking to them combined with the confirmation of the visual representations of the LAD, needed to be in place for augmentation to emerge. Rather than the LAD supporting and confirming the teacher’s prior knowledge, Kim needed to act confirmatively in relation to the relevance of the statistics, even when it was obviously not relevant as some of the students demonstratively disengaged with the data collection. This confirmation was further emphasised when Kim was confronted by the researcher who wondered what the LAD could possibly offer in terms of new insights. Rather than dismissing the value of measuring and representing well-being with red emojis, Kim confirmed their validity by arguing that ‘gut feeling’ was less significant without the numbers and statistical representations to support it. Again, the teacher’s confirmation was a key ingredient in the symmation process, while also creating a paradox where other ways of knowing in the classroom were being challenged by the LAD and were simultaneously pivotal for the co-production of the augmentation of teachers.
Concluding discussion
Amid the rapidly growing interest in AI in education, this paper demonstrates how automation or augmentation can emerge in teachers’ practices. These concepts—the automation or augmentation of teachers—are, as illustrated in this paper, co-produced with teachers, students, ideas and the material conditions, rather than being inherent affordances of AI technologies. In response to our research questions, we have illustrated how this co-production—symmation—comes into being through the enactments of teachers, students, ideas and material conditions. By doing so, the paper not only demystifies how heterogeneous and context-dependent AI technologies may play out in Swedish classrooms. It also exposes teachers’ subtle-yet-intricate, behind-the-scenes work.
Hidden work
Rather than reducing the workload of teachers or providing new insights, the added or redistributed work of AI implementation, as presented in this study, seems to reshape teachers’ practices. Teachers’ adaptations highlight how teachers must carefully tailor their teaching and constantly monitor and support their students in accommodating the AI Engine’s unpredictable ‘personalisation’. Similarly, teachers’ experimentation underscores the compliance and flexibility needed from the teachers as well as the students when acting in response to algorithmic decision-making. From this event, we can also understand that the machine-learning algorithms are not neutral but founded on particular value-driven ideas about teaching and learning that result in decisions regarding when a student should progress to another difficulty level that are not necessarily aligned with the insights of teachers or the needs of students (Selwyn, 2022b; Williamson, 2017). Teachers’ compensations and confirmations highlight how AI technologies, rather than revealing things about students and their learning that teachers otherwise would not be able to observe or know, largely depend on teachers’ implicit labour and act as ‘storytelling devices’ (Jarke & Macgilchrist, 2021). Within these stories or narratives, numerical data, pie charts and emojis are intricately woven into the fabric of teachers’ pre-existing knowledge, assuming the role of affirming or dissenting, being positive or negative, true or false, relevant or insignificant. Thus, paradoxically, teachers’ existing knowledge and discernment, as well as concrete actions to support the technology, are prerequisites for the expectations of automation and augmentation. These different enactments of symmation not only reshape teachers’ practices but also point to numerous hidden ethical dilemmas.
Hidden dilemmas
The ethics of AI related to students’ privacy, algorithmic decision transparency and teachers’ autonomy have already come under increasing scrutiny (Borenstein et al., 2021; Cerratto Pargman et al., 2021; Slade & Prinsloo, 2017). This also relates to the legal considerations regarding the introduction of AI at-scale. This introduction implies that teacher and student often contribute with unseen and unpaid work by generating data that has a commercial value for the system producers and vendors (Day et al., 2022; Selwyn, 2019b). By accentuating the hidden work of teachers in this paper, we point to a less acknowledged dilemma related to the discourse surrounding AI in education in which teachers’ workloads are expected to be reduced (automated) and teachers’ knowledge about students and their progress enhanced (augmented). This discourse builds on the premises that: (1) automation and augmentation are affordances brought by the technology; and (2) that automation and augmentation are seen as unquestionably positive improvements of education at large. Based on our findings, we suggest a more nuanced and critical approach; teachers’ efforts are essential in co-producing the illusion of automation and augmentation, and this additional work poses an ethical dilemma in itself. The empirical examples further highlight subtle and specific dilemmas related to teachers’ adaptations, experimentation, compensations, and confirmations. These dilemmas arise when colours and numerical values speak against teachers’ professional judgement, thereby risking reshaping the relationships between students and teachers (Farazouli et al., 2023; Jarke & Macgilchrist, 2021). Such dilemmas also include teachers’ devaluations of their professional judgement, accumulated through extensive embodied interactions with their students (see Bornemark, 2020), making it necessary for teachers to be the ones confirming the LAD and not vice versa. The automated decision-making of AI-based technologies also poses ethical dilemmas related to authority in the classroom and the accountability of teaching. This in turn relates to what kind of professional knowledge teachers are expected to have. All these aspects of teaching (teachers’ professional judgement, authority in the classroom and accountability) affect the dynamics within student–teacher relationships and are being challenged by the automatic decision-making of AI-based technologies.
Future directions
While the exposure of teachers’ (hidden) work in this paper may ‘crumble away’ some of the magic of AI (cf. Weizenbaum, 1966) and ignite discussions regarding teachers’ work and professional knowledge, we propose a more far-reaching conversation concerning the role of AI in Nordic education in forthcoming years. Such a conversation should engage a broader spectrum of educational researchers, teachers and educators to ensure that the envisioned future transcends industry-driven perspectives and transforms educational practices, processes and organisations in ways that are democratic, ethical and pedagogically desirable. This is particularly important with AI in education turning into a commercial multi-million-dollar industry that is rapidly impacting educational policy and practice (Komljenovic & Lee Robertson, 2017). Thus, it is likely that these multifaceted technologies will, to an even greater extent than today, involve teachers and impact their work in unforeseen and potentially harmful ways.
Our findings are based on two distinct contextually bound ethnographic studies related to a research and innovation project and a co-design process, in which a relatively small group of teachers willingly tried out the specific technologies in teaching practice despite encountering technical challenges. By acknowledging the specificity and size of the study, we underscore the importance of additional educational research related to fine-grained, hard-to-capture insights into AI in situated classroom practice. In this context, the concept of symmation can be productive to further explore and ‘break the magic’ of the complex and messy enactments of teachers and AI technologies.
References
- Akata, Z., Balliet, D., De Rijke, M., Dignum, F., Dignum, V., Eiben, G., Fokkens, A., Grossi, D., Hindriks, K., Hoos, H., Hung, H., Jonker, C., Monz, C., Neerincx, M., Oliehoek, F., Prakken, H., Schlobach, S., Van Der Gaag, L., Van Harmelen, F., … Welling, M. (2020). A research agenda for hybrid intelligence: Augmenting human intellect with collaborative, adaptive, responsible, and explainable artificial intelligence. Computer, 53(8), 18–28. https://doi.org/10.1109/MC.2020.2996587
- Bearman, M., & Ajjawi, R. (2023). Learning to work with the black box: Pedagogy for a world with artificial intelligence. British Journal of Educational Technology, 54(5), 1160–1173. https://doi.org/10.1111/bjet.13337
- Borenstein, J., Grodzinsky, F. S., Howard, A., Miller, K. W., & Wolf, M. J. (2021). AI ethics: A long history and a recent burst of attention. Computer, 54(1), 96–102. https://doi.org/10.1109/MC.2020.3034950
- Bornemark, J. (2020). Horisonten finns alltid kvar: Om det bortglömda omdömet [The horizon is always there: On the forgotten reputation]. Volante
- Buckingham Shum, S. J., & Luckin, R. (2019). Learning analytics and AI: Politics, pedagogy and practices. British Journal of Educational Technology, 50(6), 2785–2793. https://doi.org/10.1111/bjet.12880
- Cerratto Pargman, T., & McGrath, C. (2021). Mapping the ethics of learning analytics in higher education: A systematic literature review of empirical research. Journal of Learning Analytics, 8(2), 123–139. https://doi.org/10.18608/jla.2021.1
- Cukurova, M., Kent, C., & Luckin, R. (2019). Artificial intelligence and multimodal data in the service of human decision-making: A case study in debate tutoring. British Journal of Educational Technology, 50(6), 3032–3046.
- Day, E., Pothong, K., Atabey, A., & Livingstone, S. (2022). Who controls children’s education data? A socio-legal analysis of the UK governance regimes for schools and EdTech. Learning, Media and Technology, 1–15. https://doi.org/10.1080/17439884.2022.2152838
- Dellermann, D., Ebel, P., Söllner, M., & Leimeister, J. M. (2019). Hybrid intelligence. Business & Information Systems Engineering, 61(5), 637–643. https://doi.org/10.1007/s12599-019-00595-2
- Dépelteau, F. (2013). What is the direction of the “relational turn”? In C. Powell, & F. Dépelteau (Eds.), Conceptualizing relational sociology (pp. 163–185). Springer.
- du Boulay, B. (2019). Escape from the Skinner box: The case for contemporary intelligent learning environments. British Journal of Educational Technology, 50(6), 2902–2919.
- du Boulay, B. (2022). Artificial intelligence in education and ethics. In O. Zawacki-Richter, & I. Jung (Eds.), Handbook of open, distance and digital education (pp. 1–16). Springer.
- Farazouli, A., Cerratto-Pargman, T., Bolander-Laksov, K., & McGrath, C. (2023). Hello GPT! Goodbye home examination? An exploratory study of AI chatbots impact on university teachers’ assessment practices. Assessment & Evaluation in Higher Education, 1–13.
- Fenwick, T., & Edwards, R. (2010). Actor-network theory in education. Routledge.
- Fenwick, T., & Landri, P. (2012). Materialities, textures and pedagogies: Socio-material assemblages in education. Pedagogy, Culture & Society, 20(1), 1–7. https://doi.org/10.1080/14681366.2012.649421
- Ferguson, R., & Clow, D. (2017). Where is the evidence? A call to action for learning analytics. LAK ‘17 Proceedings of the Seventh International Learning Analytics & Knowledge Conference. 13–17.
- Holmes, W., Bialik, M., & Fadel, C. (2020). Artificial intelligence in education. Promise and implications for teaching and learning. Center for Curriculum Redesign.
- Holmes, W., & Tuomi, I. (2022). State of the art and practice in AI in education. European Journal of Education, 57(4), 542–570. https://doi.org/10.1111/ejed.12533
- Jackson, A. Y., & Mazzei, L. A. (2013). Plugging one text into another: Thinking with theory in qualitative research. Qualitative Inquiry, 19(4), 261–271.
- Jarke, J., & Macgilchrist, F. (2021). Dashboard stories: How narratives told by predictive analytics reconfigure roles, risk and sociality in education. Big Data & Society, 8(1), 20539517211025561.
- Knox, J., Williamson, B., & Bayne, S. (2020). Machine behaviourism: Future visions of ‘learnification’and ‘datafication’across humans and digital technologies. Learning, Media and Technology, 45(1), 31–45.
- Komljenovic, J., & Lee Robertson, S. (2017). Making global education markets and trade. Globalisation, Societies and Education, 15(3), 289–295. https://doi.org/10.1080/14767724.2017.1330140
- Lather, P., & St. Pierre, E. A. (2013). Post-qualitative research. International Journal of Qualitative Studies in Education, 26(6), 629–633.
- Latour, B. (2007). Reassembling the social: An introduction to actor-network-theory. Oxford University Press.
- Law, J. (2004). After method: Mess in social science research. Routledge.
- Lupton, D., & Williamson, B. (2017). The datafied child: The dataveillance of children and implications for their rights. New Media & Society, 19(5), 780–794.
- MacLure, M. (2013). The wonder of data. Cultural Studies? Critical Methodologies, 13(4), 228–232.
- Matcha, W., Uzir, N. A., Gasevic, D., & Pardo, A. (2020). A systematic review of empirical studies on learning analytics dashboards: A self-regulated learning perspective. IEEE Transactions on Learning Technologies, 13(2), 226–245. https://doi.org/10.1109/TLT.2019.2916802
- Mazzei, L. A. (2013). A voice without organs: Interviewing in posthumanist research. International Journal of Qualitative Studies in Education, 26(6), 732–740. https://doi.org/10.1080/09518398.2013.788761
- Molenaar, I. (2022). Towards hybrid human-AI learning technologies. European Journal of Education, 57(4), 632–645. https://doi.org/10.1111/ejed.12527
- Molenaar, I., & Knoop-van Campen, C. A. N. (2019). How teachers make dashboard information actionable. IEEE Transactions on Learning Technologies, 12(3), 347–355. https://doi.org/10.1109/TLT.2018.2851585
- Park, Y., & Jo, I.-H. (2019). Factors that affect the success of learning analytics dashboards. Educational Technology Research and Development, 67(6), 1547–1571. https://doi.org/10.1007/s11423-019-09693-0
- Perrotta, C., Gulson, K. N., Williamson, B., & Witzenberger, K. (2021). Automation, APIs and the distributed labour of platform pedagogies in Google Classroom. Critical Studies in Education, 62(1), 97–113.
- Perrotta, C., & Selwyn, N. (2020). Deep learning goes to school: Toward a relational understanding of AI in education. Learning, Media and Technology, 45(3), 251–269. https://doi.org/10.1080/17439884.2020.1686017
- Perrotta, C., & Williamson, B. (2018). The social life of learning analytics: Cluster analysis and the ‘performance’ of algorithmic education. Learning, Media and Technology, 43(1), 3–16. https://doi.org/10.1080/17439884.2016.1182927
- Petersmann, M.-C. Sympoietic thinking and Earth System Law: The Earth, its subjects and the law. Earth System Governance, 9, 100114.
- Popenici, S. A. D., & Kerr, S. (2017). Exploring the impact of artificial intelligence on teaching and learning in higher education. Research and Practice in Technology Enhanced Learning, 12(1), 22. https://doi.org/10.1186/s41039-017-0062-8
- Schwendimann, B. A., Rodriguez-Triana, M. J., Vozniuk, A., Prieto, L. P., Boroujeni, M. S., Holzer, A., Gillet, D., & Dillenbourg, P. (2017). Perceiving learning at a glance: A systematic literature review of learning dashboard research. IEEE Transactions on Learning Technologies, 10(1), 30–41. https://doi.org/10.1109/TLT.2016.2599522
- Selwyn, N. (2019a). Should robots replace teachers?: AI and the future of education. John Wiley & Sons.
- Selwyn, N. (2019b). What’s the problem with learning analytics? Journal of Learning Analytics, 6(3), 11–19.
- Selwyn, N. (2022a). Less work for teacher? The ironies of automated decision-making in schools. In S. PInk, M. Berg, D. Lupton, & M. Ruckenstein (Eds.), Everyday automation (pp. 73–86). Routledge.
- Selwyn, N. (2022b). The future of AI and education: Some cautionary notes. European Journal of Education, 57(4), 620–631. https://doi.org/10.1111/ejed.12532
- Selwyn, N., Hillman, T., Bergviken-Rensfeldt, A., & Perrotta, C. (2023). Making sense of the digital automation of education. Postdigital Science and Education, 5(1), 1–14.
- Siemens, G. (2013). Learning analytics: The emergence of a discipline. American Behavioral Scientist, 57(10), 1380–1400.
- Slade, S. & Prinsloo, P. (2017). Ethics and learning analytics: Charting the (un)charted. In C. Lang, G. Siemens, A. Wise, & D. Gašević (Eds.), Handbook of learning analytics (pp. 49–57). Solar. https://doi.org/10.18608/hla17.004
- Sperling, K., Stenliden, L., Nissen, J., & Heintz, F. (2022). Still w(AI)ting for the automation of teaching: An exploration of machine learning in Swedish primary education using Actor-Network Theory. European Journal of Education, 57(4), 584–600. https://doi.org/10.1111/ejed.12526
- Sperling, K., Stenliden, L., Nissen, J. et al. (2023). Behind the scenes of co-designing AI and LA in K-12 education. Postdigital Science and Education. https://doi.org/10.1007/s42438-023-00417-5
- Stafford, B. M. (2001). Revealing technologies/magical domains. In B. Stafford & F. Terpak (Eds.), Devices of wonder: From the world in a box to images on a screen (pp. 1–142). Getty Research Institute.
- St. Pierre, E. A., & Jackson, A. Y. (2014). Qualitative data analysis after coding. Qualitative Inquiry, 20(6), 715–719. https://doi.org/10.1177/1077800414532435
- Tuomi, I., Cachia, R., & Villar Onrubia, D. (2023). On the futures of technology in education: Emerging trends and policy implications. Publications Office of the European Union. https://doi.org/10.2760/079734, JRC134308
- Verbert, K., Govaerts, S., Duval, E., Santos, J. L., Van Assche, F., Parra, G., & Klerkx, J. (2014). Learning dashboards: An overview and future research opportunities. Personal and Ubiquitous Computing, 18(6), 1499–1514.
- Viberg, O., Hatakka, M., Bälter, O., & Mavroudi, A. (2018). The current landscape of learning analytics in higher education. Computers in Human Behavior, 89, 98–110.
- Wagener-Böck, N., Macgilchrist, F., Rabenstein, K., & Bock, A. (2023). From automation to symmation: Ethnographic perspectives on what happens in front of the screen. Postdigital Science and Education, 5(1), 136–151. https://doi.org/10.1007/s42438-022-00350-z
- Weizenbaum, J. (1966). ELIZA—a computer program for the study of natural language communication between man and machine. Communications of the ACM, 9(1), 36–45. https://doi.org/10.1145/365153.365168
- Williamson, B. (2017). Big data in education: The digital future of learning, policy and practice. Sage.
- Williamson, B., & Eynon, R. (2020). Historical threads, missing links, and future directions in AI in education. Learning, Media and Technology, 45(3), 223–235.
- Zawacki-Richter, O., Marín, V. I., Bond, M., & Gouverneur, F. (2019). Systematic review of research on artificial intelligence applications in higher education–where are the educators? International Journal of Educational Technology in Higher Education, 16(1), 1–27.
Fotnoter
- 1. All names are pseudonyms.